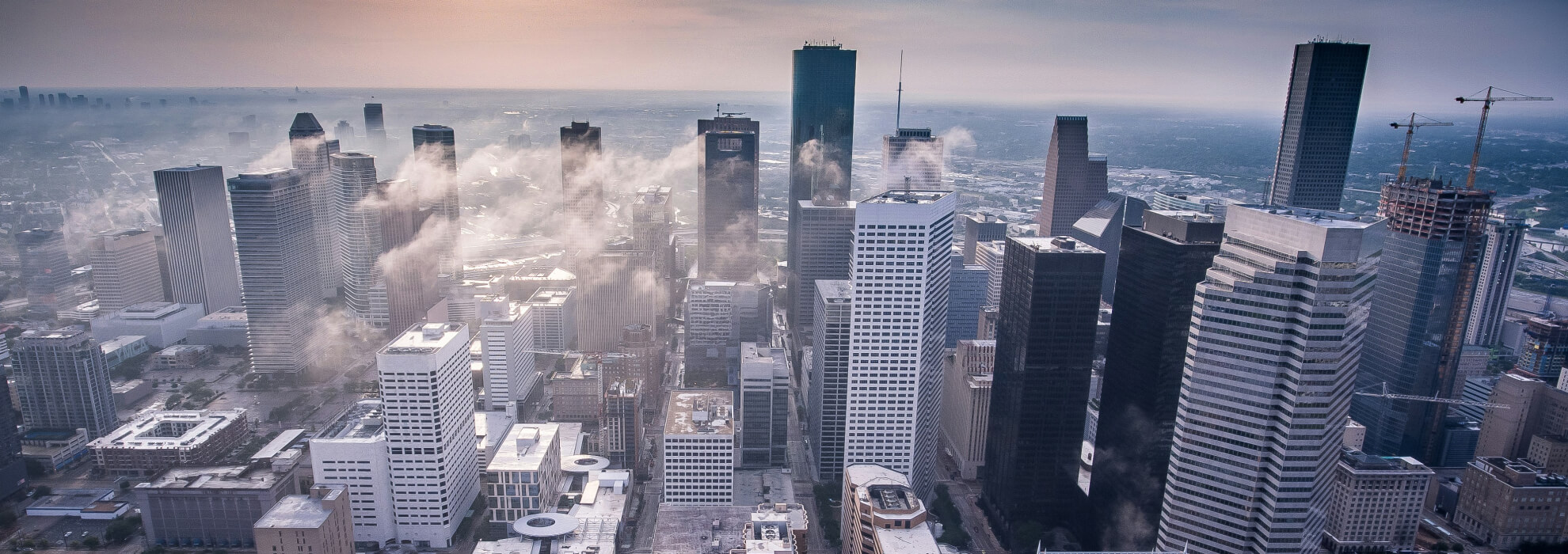
Watch short for this article (5 slides)
From Skywatching to Supercomputers: The Evolution and Future of Weather Forecasting
Checking a weather forecast on a smartphone - a seemingly simple act involving icons and numbers - belies centuries of scientific struggle, technological breakthroughs, and computational evolution. Predicting the weather, a factor profoundly influencing everything from agriculture to travel to daily safety, has driven human ingenuity for millennia. From ancient sky-watchers interpreting cloud patterns to sophisticated AI algorithms processing petabytes of data, the journey of weather forecasting is a remarkable story of humanity's quest to understand and anticipate the complex dance of the atmosphere. How did we get here, and what does the future hold for predicting rain, shine, and storms?
Early Foundations: Observation, Instruments, and Networks
Folk Wisdom and the Limits of Observation
For most of human history, weather prediction relied on empirical observation passed down through generations. Farmers noted the behavior of animals, sailors scrutinized cloud types and wind shifts, and communities developed weather lore - sayings linking certain signs (like a "red sky at night") to upcoming conditions. While often based on valid local correlations, these methods lacked quantitative rigor, predictive range, and the ability to understand the larger atmospheric systems driving local weather.
The Instrumental Revolution: Quantifying the Atmosphere
The Scientific Revolution brought instruments that allowed for objective measurement of atmospheric variables, marking a pivotal shift. Key inventions included:
- The Barometer (c. 1643): Invented by Evangelista Torricelli, this device measured atmospheric pressure. Early mercury barometers worked by balancing the weight of the atmosphere against a column of mercury in a sealed tube; rising pressure indicated fair weather approaching, while falling pressure often signaled impending storms. This provided the first truly quantitative tool for anticipating weather changes.
- The Thermometer (evolving through 17th-18th centuries): Allowed for precise measurement of air temperature, crucial for understanding air masses and fronts.
- The Hygrometer (evolving through 17th-18th centuries): Measured humidity, another key factor in cloud formation and precipitation potential.
These instruments moved forecasting from subjective interpretation towards objective data collection.
Connecting the Dots: The Telegraph and Synoptic Meteorology
The invention of the electrical telegraph in the mid-19th century was revolutionary for meteorology. For the first time, observations of pressure, temperature, wind, and weather conditions from widely separated locations could be collected rapidly at a central point. This enabled the creation of synoptic weather maps - maps showing weather conditions over a large area at a single point in time.
By analyzing these maps, meteorologists could identify and track large-scale weather systems like high and low-pressure areas, fronts, and storms. This marked the birth of modern synoptic meteorology and allowed for the first scientifically-based forecasts covering larger regions and longer timeframes (initially perhaps 12-24 hours). Pioneers like Admiral Robert FitzRoy in the UK used this system to issue the first public storm warnings in the 1860s.
The Birth of Numerical Weather Prediction (NWP)
The Theoretical Dream: Physics and Mathematics Take Center Stage
At the turn of the 20th century, scientists realized that the atmosphere behaved according to the laws of physics and fluid dynamics. Norwegian physicist Vilhelm Bjerknes and his colleagues proposed that weather forecasting could, in principle, be treated as an initial value problem in mathematical physics. If one knew the current state of the atmosphere (the initial conditions) and the physical laws governing its motion (expressed as differential equations - the "primitive equations"), one could calculate its future state.
British mathematician and meteorologist Lewis Fry Richardson attempted the first practical numerical forecast in the 1920s. He spent weeks performing painstaking manual calculations using Bjerknes's equations to predict weather changes over a small part of Europe for just six hours ahead. His published work, "Weather Prediction by Numerical Process" (1922), was visionary but ultimately resulted in a wildly inaccurate forecast. The reasons were twofold:
- Computational Limits: Manual calculations were far too slow. Richardson famously estimated needing 64,000 human "computers" working simultaneously just to keep pace with the weather.
- Scientific Challenges: Inaccurate initial data and imperfections in the understanding and representation of atmospheric physics (particularly small-scale processes) led to errors rapidly amplifying in the calculations - an early hint of the atmosphere's chaotic nature later described by Edward Lorenz.
The Computer Revolution: Making NWP Viable
Richardson's dream remained dormant until the advent of electronic computers after World War II. In the early 1950s, a team led by meteorologist Jule Charney and mathematician John von Neumann used one of the earliest computers, the ENIAC (Electronic Numerical Integrator and Computer), to perform the first successful numerical weather forecast. For the first time, the complex calculations could be completed significantly faster than the weather itself evolved, proving the practical feasibility of NWP.
The advent of the computer age transformed meteorology from a largely descriptive science into a quantitative, predictive powerhouse. It finally provided the tool needed to solve the complex equations governing atmospheric flow. - Atmospheric Science Historian perspective.
Refining the Forecast: Satellites, Models, and Global Collaboration
The latter half of the 20th century saw continuous refinement of NWP, driven by better data, more powerful computers, and improved models.
The View from Above: The Satellite Era
The launch of the first weather satellite, TIROS-1, by NASA in 1960 opened a new window onto the atmosphere. Satellites provided unprecedented global coverage, especially over oceans and remote land areas where ground-based observations were scarce. They delivered crucial data, including:
- Visible and Infrared Imagery: Allowing meteorologists to track cloud patterns, storms, cyclones, and fronts globally.
- Atmospheric Soundings: Measuring temperature and humidity profiles at different altitudes.
- Sea Surface Temperatures: Critical for understanding ocean-atmosphere interactions and hurricane development.
- Wind Data: Derived from tracking cloud movements or measuring microwave scattering from the ocean surface.
This influx of global data dramatically improved the accuracy of the initial conditions fed into NWP models.
Better Models, Better Forecasts
Improvements in NWP involved several key areas:
- Increased Resolution: Early models had grid resolutions of hundreds of kilometers. Modern global models run at resolutions around 10-15 kilometers, with regional models achieving even finer scales (1-4 km). Higher resolution allows models to better represent smaller-scale weather features and terrain effects.
- Improved Physics (Parameterization): Models must approximate processes occurring at scales smaller than the grid resolution (like cloud formation, radiation transfer, turbulence). Developing more accurate "parameterization schemes" significantly improved forecast skill.
- Data Assimilation: Sophisticated techniques were developed to optimally combine observational data (from satellites, ground stations, weather balloons, aircraft) with the model's previous forecast state to create the best possible analysis of current conditions (the "initial state").
- Ensemble Forecasting: Recognizing the atmosphere's chaotic nature (the "butterfly effect" described by Edward Lorenz, where small errors in initial conditions grow rapidly), ensemble forecasting was developed. Instead of running one forecast, multiple forecasts ("ensemble members") are run with slightly perturbed initial conditions or different model physics. The spread among the ensemble members provides crucial information about forecast uncertainty and the probability of different weather outcomes.
Global Cooperation
Weather knows no borders. International collaboration became essential. Organizations like the World Meteorological Organization (WMO) facilitate data sharing. Major centers like the European Centre for Medium-Range Weather Forecasts (ECMWF), the US National Centers for Environmental Prediction (NCEP), and others run sophisticated global models, pooling resources and expertise to produce forecasts used worldwide.
The AI Transformation: Learning from Data
While NWP based on physics equations remains the bedrock of modern forecasting, Artificial Intelligence (AI) and Machine Learning (ML) are rapidly emerging as powerful complementary tools.
Addressing NWP Limitations
Despite immense progress, NWP faces challenges: running high-resolution global models is computationally extremely expensive, requiring massive supercomputers, and parameterization schemes for complex processes like cloud microphysics remain imperfect.
How AI is Changing the Game
AI excels at identifying complex patterns in vast datasets. In meteorology, AI is being applied in several ways:
- Data-Driven Forecasting Models: AI models like Google DeepMind's GraphCast and Pangu-Weather (Huawei), or NVIDIA's FourCastNet, learn the evolution of weather patterns directly from decades of historical weather data and analyses, rather than explicitly solving physics equations. Once trained (which is computationally intensive), these models can often produce forecasts significantly faster than traditional NWP models on less powerful hardware. They have shown remarkable skill, sometimes rivaling or even exceeding top NWP models for certain forecast ranges and variables. (Source: Google DeepMind - GraphCast)
- Nowcasting: AI is particularly effective for very short-range forecasts (0-2 hours), known as nowcasting. By analyzing real-time radar and satellite imagery, AI models can predict the immediate movement and intensity of rainfall and thunderstorms with high precision, crucial for flash flood warnings and aviation.
- Post-Processing/Improving NWP Output: AI can be used to correct systematic biases in NWP model output or downscale forecasts to specific locations, improving accuracy by learning local effects not captured by the coarser model grid.
- Data Assimilation & Quality Control: AI techniques can help process and quality-control the massive amounts of observational data used to initialize NWP models.
The current trend is towards hybrid approaches, combining the strengths of physics-based NWP with data-driven AI insights.
Impacts on Society: From Daily Convenience to Critical Decisions
The advancements in weather forecasting have permeated nearly every aspect of modern life:
- Public Safety: Improved warnings for severe weather (tornadoes, hurricanes, floods, heatwaves) provide crucial lead time for evacuations and preparedness, saving countless lives. Hurricane track forecasts, for example, have improved dramatically, reducing the size of evacuation zones.
- Agriculture: Precision forecasts inform decisions on planting, irrigation, fertilization, pest control, and harvesting, optimizing yields and resource use.
- Aviation: Accurate forecasts of wind, turbulence, icing, and thunderstorms enhance flight safety, optimize routes for fuel efficiency, and reduce delays.
- Energy Sector: Forecasts predict energy demand (heating/cooling) and the output of renewable sources like wind and solar power, crucial for grid management.
- Transportation and Logistics: Shipping routes are optimized to avoid storms; road maintenance crews prepare for snow or ice; supply chains anticipate weather-related disruptions.
- Daily Life: Accessible forecasts via apps and websites allow individuals to plan daily activities, travel, and recreation with greater confidence.
The Horizon: Future Directions and Challenges
- Hyperlocal and High-Resolution: Continued increases in computing power and model sophistication will enable forecasts at neighborhood scales (sub-kilometer resolution), capturing highly localized phenomena like urban heat islands or complex terrain effects.
- Seamless AI-NWP Integration: Developing robust hybrid models that leverage the speed and pattern recognition of AI with the physical consistency of NWP is a major focus.
- Probabilistic Forecasting Dominance: Increasing emphasis on communicating forecast uncertainty using ensemble data and probabilistic forecasts ("X% chance of rain") rather than single deterministic predictions.
- Quantum Computing?: While still speculative, quantum computing could potentially revolutionize aspects of NWP or AI model training in the long term by tackling currently intractable computational problems.
- Climate Change Impact: A changing climate introduces new challenges, potentially altering established weather patterns and increasing the frequency and intensity of extreme events, making accurate forecasting even more critical but potentially more difficult.
- The Human Element: Despite technological advances, skilled human meteorologists remain essential for interpreting complex model output, communicating forecasts and risks effectively, making critical decisions during high-impact events, and handling situations beyond the training data of AI models.
Conclusion: An Ongoing Scientific Endeavor
From rudimentary observations to global satellite networks feeding powerful AI models, weather forecasting has undergone a profound transformation. The journey, marked by ingenious instrumentation, theoretical breakthroughs, computational revolutions, and international collaboration, allows us today to anticipate atmospheric behavior with an accuracy unimaginable just generations ago. While the inherent chaos of the atmosphere means perfect prediction remains an elusive goal, the relentless pace of scientific and technological progress continues to push the boundaries.
The fusion of physics-based modeling and data-driven AI promises even greater accuracy, speed, and localization in the future. As we face the growing challenges of a changing climate, the ongoing evolution of weather forecasting will be more crucial than ever, providing the foresight needed to navigate our dynamic atmosphere safely and sustainably.