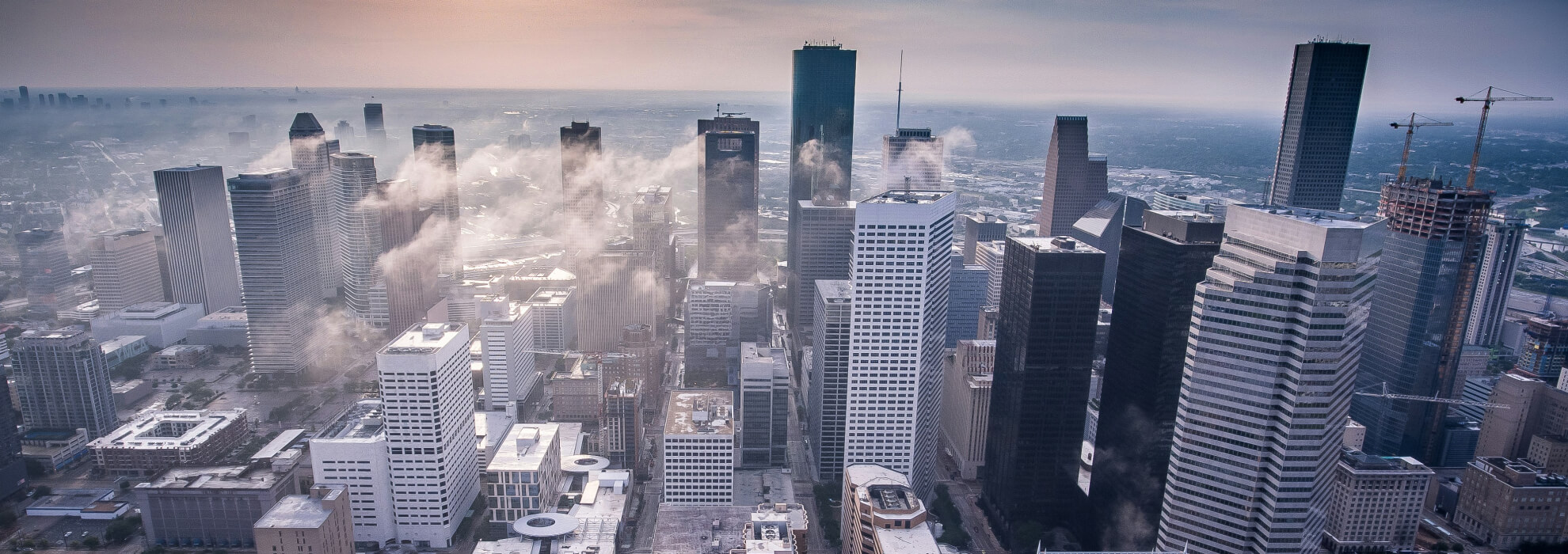
Watch short for this article (5 slides)
The AI Sustainability Paradox: Powering Progress, Consuming the Planet?
Artificial intelligence (AI) is rapidly transforming industries and reshaping society, offering unprecedented capabilities for optimization, discovery, and problem-solving. Its potential applications in tackling the climate crisis – from optimizing renewable energy grids to accelerating the discovery of sustainable materials – are immense. However, this promise is shadowed by a growing concern: the significant environmental footprint of AI itself. The energy-hungry data centers training vast models and the resource-intensive hardware powering them contribute substantially to global carbon emissions and resource depletion. This paradox – AI as both a potential savior and a contributor to the climate problem – demands careful examination and conscious choices about how we develop and deploy this powerful technology.
Unpacking AI's Environmental Footprint: The Hidden Costs of Computation
The impressive capabilities of modern AI, particularly large language models (LLMs) and deep learning systems, come at a significant environmental cost, primarily driven by:
1. Energy-Intensive Data Centers
AI computations, both for training models and running them (inference), require massive amounts of processing power housed in specialized data centers.
- Electricity Consumption: Data centers globally already consume an estimated 1-2% of the world's electricity, and the rapid growth of AI is significantly increasing this demand. Training a single large-scale AI model can consume gigawatt-hours (GWh) of electricity. For context, a widely cited 2019 study estimated that training a specific large deep-learning model for natural language processing could emit over 284 tonnes of carbon dioxide equivalent – roughly five times the lifetime emissions of an average American car, including its manufacture. (Strubell et al., 2019 - Energy and Policy Considerations for Deep Learning). While efficiency is improving, the scale of models is also growing.
- Cooling Requirements & Water Usage: Servers generate immense heat, necessitating powerful cooling systems (often water-based) which consume additional energy and significant amounts of water. The Water Usage Effectiveness (WUE) is becoming as critical a metric as Power Usage Effectiveness (PUE).
- Geographic Concentration: Data centers are often clustered in specific regions, potentially straining local energy grids and water resources.
2. The Energy Cost of Model Training vs. Inference
It's important to distinguish between the two main phases of AI computation:
- Training: This initial phase involves feeding vast datasets into AI models to teach them patterns and capabilities. It is computationally extremely intensive, requiring prolonged runs on powerful hardware (like GPUs and TPUs), consuming enormous amounts of energy in concentrated bursts.
- Inference: This is the phase where a trained model is used to make predictions, generate text, analyze data, etc. While a single inference query uses far less energy than training, the sheer volume of queries for popular AI services means the cumulative energy consumption of inference globally can outweigh that of training over the model's lifetime.
3. Hardware Lifecycle: From Manufacturing to E-Waste
The environmental impact isn't just about electricity consumption during operation:
- Manufacturing Emissions: Producing the specialized hardware required for AI – Graphics Processing Units (GPUs), Tensor Processing Units (TPUs), advanced semiconductors – is energy- and resource-intensive. This involves mining rare earth minerals, significant water usage, complex supply chains, and associated carbon emissions (often referred to as "embodied carbon").
- Rapid Obsolescence & E-Waste: The fast pace of AI development drives frequent hardware upgrades, leading to a shorter lifespan for expensive components and contributing significantly to the growing problem of electronic waste (e-waste), which contains hazardous materials and is challenging to recycle.
4. Cloud Computing Abstraction
While cloud services make AI accessible, they abstract the underlying infrastructure. Using cloud-based AI still relies on massive data centers operated by companies like Amazon (AWS), Google (GCP), and Microsoft (Azure), contributing to the overall energy and resource footprint, even if the end-user doesn't see the direct energy bill.
AI as a Tool for Sustainability: Harnessing Intelligence for a Greener Future
Despite its environmental costs, AI offers powerful capabilities to address climate change and sustainability challenges across various sectors:
1. Optimizing Energy Systems
- Grid Modernization: AI algorithms can forecast energy demand and renewable energy generation (solar/wind) with greater accuracy, enabling better grid balancing, reducing reliance on fossil fuel peaker plants, and facilitating higher penetration of intermittent renewables.
- Energy Efficiency: AI can optimize energy use in buildings (smart thermostats, lighting control), industrial processes, and transportation logistics, significantly reducing waste. Google, for example, reported using AI to reduce the energy needed for cooling its data centers significantly. (DeepMind Blog on Data Center Cooling)
2. Accelerating Climate Science and Environmental Monitoring
- Climate Modeling: AI can analyze vast climate datasets and accelerate complex climate simulations, improving the accuracy and speed of climate change projections.
- Remote Sensing Analysis: Machine learning excels at analyzing satellite and drone imagery to monitor deforestation, track greenhouse gas emissions sources (like methane leaks), monitor ice melt and sea-level rise, assess biodiversity, and detect pollution events in near real-time.
3. Enhancing Resource Management and Circular Economy
- Precision Agriculture: AI can analyze soil conditions, weather patterns, and crop health (via sensors/imagery) to optimize irrigation, fertilizer, and pesticide use, reducing resource consumption and environmental runoff.
- Supply Chain Optimization: AI algorithms can optimize logistics routes, reduce transportation emissions, improve inventory management to minimize waste, and enhance predictive maintenance for industrial equipment.
- Materials Science: AI is being used to accelerate the discovery and development of new sustainable materials, such as better catalysts for green hydrogen production, more efficient solar cells, or materials for advanced batteries.
4. Improving Carbon Capture and Removal
- AI can help optimize the design and operation of Carbon Capture, Utilization, and Storage (CCUS) technologies, simulate geological storage sites, and potentially identify novel materials or methods for direct air capture (DAC).
Weighing the Scales: Impact vs. Benefit
Directly comparing AI's environmental footprint with its potential sustainability benefits is complex. The negative impacts (energy use, emissions, e-waste) are relatively direct consequences of its operation and manufacture. The positive impacts are often indirect, realized through the application of AI in other sectors to improve efficiency or enable new solutions. A crucial factor is *how* AI is deployed – using AI for energy optimization yields clear benefits, while using it for energy-intensive tasks with limited societal value (e.g., generating spam) exacerbates the problem.
AI's Environmental Cost Category | Corresponding Potential AI Benefit Category | Example of Benefit |
---|---|---|
High energy use (Data Centers, Training, Inference) | Energy System Optimization & Efficiency | AI managing smart grids to integrate more renewables; optimizing building HVAC systems. |
Greenhouse Gas Emissions (Direct & Embodied) | Climate Science & Carbon Management | AI accelerating climate models; optimizing carbon capture processes; tracking emissions via satellite. |
Hardware Resource Consumption & E-Waste | Resource Management & Circular Economy | AI optimizing supply chains to reduce material waste; designing more durable products; precision agriculture reducing resource inputs. |
Water Consumption (Data Center Cooling) | Water Resource Management | AI optimizing irrigation systems; improving water quality monitoring and leak detection. |
Navigating the Paradox: Towards Sustainable AI
Addressing AI's environmental impact while harnessing its benefits requires a conscious and multi-faceted approach from researchers, developers, policymakers, and users:
- Develop Energy-Efficient AI:
- Algorithmic Efficiency: Researching and implementing more efficient model architectures, techniques like pruning (removing unnecessary model parts), quantization (using less precise computations), knowledge distillation (training smaller models from larger ones), and selecting appropriate model sizes for the task.
- Hardware Efficiency: Designing and utilizing more energy-efficient hardware specifically for AI tasks (e.g., TPUs, neuromorphic chips).
- Federated Learning: Training models directly on end-user devices or local servers without centralizing sensitive data, potentially reducing data transmission and central server load.
- Power AI with Renewable Energy: Siting data centers strategically near renewable energy sources, investing in direct renewable energy generation, or purchasing high-quality renewable energy credits (RECs) and power purchase agreements (PPAs). Transparency regarding the energy sources used for training and inference is key.
- Measure and Report AI's Footprint: Developing standardized methodologies and tools for measuring the energy consumption and carbon footprint across the entire AI lifecycle (from hardware manufacturing to model training and inference). Promoting transparency and corporate reporting on these metrics.
- Optimize Workloads and Usage: Critically evaluating whether complex, energy-intensive AI models are necessary for a given task; implementing techniques like load scheduling to run large training jobs during periods of high renewable energy availability.
- Promote Hardware Longevity and Circularity: Designing hardware for durability, repairability, and recyclability; developing better e-waste management systems.
- Prioritize High-Impact Applications: Focusing AI development and deployment efforts on applications with significant potential for positive environmental and societal impact, rather than purely commercial or trivial uses.
Conclusion: Shaping AI for a Sustainable Future
Artificial intelligence presents a profound duality in the face of the climate crisis. Its development and operation consume significant energy and resources, adding to the environmental burden. Yet, its unique capabilities offer powerful tools to accelerate climate mitigation and adaptation efforts across virtually every sector. The path forward lies not in abandoning AI, but in pursuing its development and deployment with a strong commitment to sustainability. This requires a concerted effort from the tech industry, researchers, policymakers, and users to prioritize energy efficiency, invest in renewable energy, demand transparency, foster innovation in "green AI" practices, and consciously direct this transformative technology towards solving our most pressing environmental challenges. Only by addressing AI's footprint while maximizing its potential for good can we ensure it becomes a net positive force for a sustainable planet.